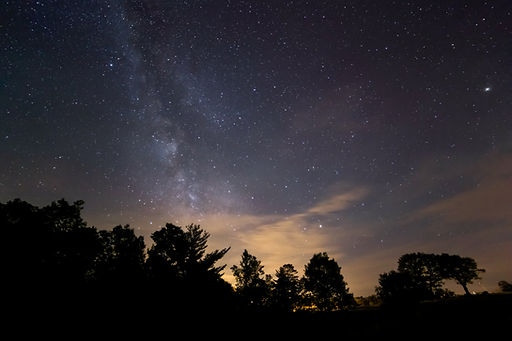
Liqiang Nie, Meng Liu, and Xuemeng Song
Multi-Modal Transductive Learning in Chapter 3.5
Our proposed transductive multi-modal learning model, which is designed to find the optimal latent common space, unifying and preserving information from different modalities, whereby the popularity of micro-videos can be better separated. The code can be download here.

Multi-Modal Consistent Learning in Chapter 4.4
We present a novel tree-guided multi-task multi-modal learning method to label the bite-sized video clips with venue categories. This model is capable of learning a common feature space from multiple and heterogonous modalities, which preserves the information of each modality via disagreement penalty. The code can be download here.

Multi-Modal Complementary Learning in Chapter 4.5
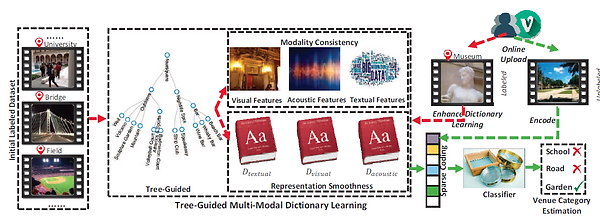
We present a novel structure-guided multi-modal dictionary learning framework for micro-video organization. This framework co-regularizes the hierarchical smoothness and structure consistency within a unified model to learn the high-level sparse representations of micro videos. Considering the timeliness and limited training samples, an online learning algorithm is developed to efficiently and incrementally strengthen the learning performance. The code can be download here.
Multi-Modal Cooperative Learning in Chapter 4.6
In this model, we introduced a novel relation-aware attention mechanism to split the consistent information from the complementary one. Following that, we integrated the consistent information to learn an enhanced consistent vector and supplemented the complementary information to enrich this enhanced vector. To learn a discriminative representation from this richer information, we devised an attention network to score the features. The code can be download here.
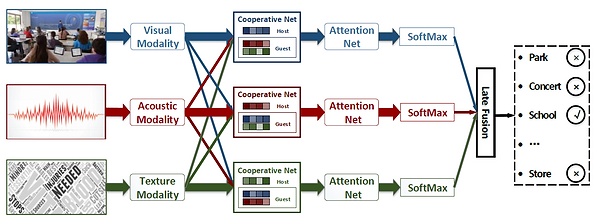
Deep Multi-Modal Transfer Learning in Chapter 5.5

We present a deep parallel sequence with sparse constraint approach to categorizing venues of micro-videos. This approach is able to jointly model the sequential structures of different modalities and sparse concept-level representations at the same time. The code can be download here.
Multi-Modal Sequential Learning in Chapter 6.4
We present a temporal graph-based LSTM model to intelligently route micro-videos to the target users. To capture the users’ dynamic and diverse interest, we encode their historical interaction sequence into a temporal graph and then design a novel temporal graph-based LSTM to model it. As different interactions reflect different degrees of interest, we build a multi-level interest modeling layer to enhance users’ interest representation. Moreover, our model extracts uninterested information from true negative samples to improve the recommendation performance. The code can be download here.
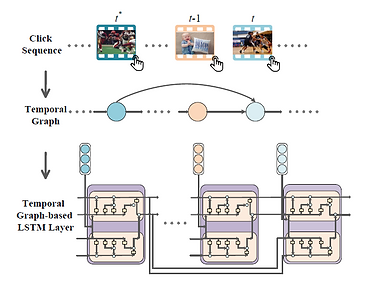